DM Control¶
DeepMind Control¶
DM Control is a framework for physics-based simulation and reinforcement learning environments using the MuJoCo physics engine.
Shimmy provides compatibility wrappers to convert Control Suite environments and custom Locomotion environments to Gymnasium.
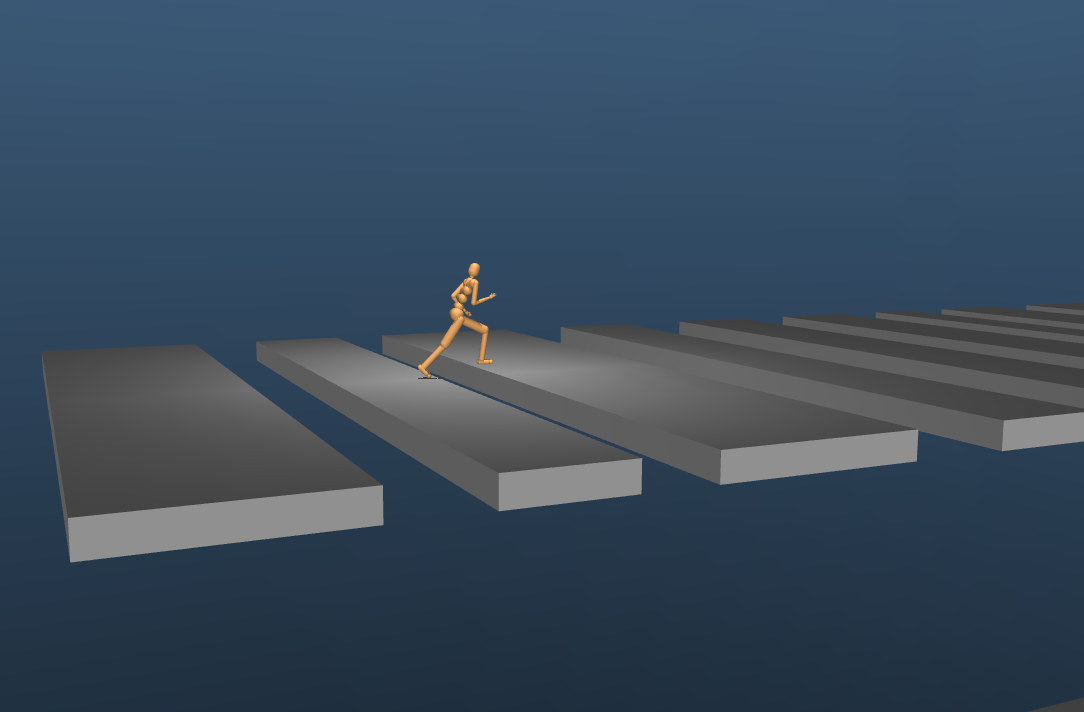
Installation¶
To install shimmy
and required dependencies:
pip install shimmy[dm-control]
We also provide a Dockerfile for reproducibility and cross-platform compatibility:
curl https://raw.githubusercontent.com/Farama-Foundation/Shimmy/main/bin/dm_control.Dockerfile | docker build -t dm_control -f - . && docker run -it dm_control
Usage¶
Load a dm_control
environment:
import gymnasium as gym
env = gym.make("dm_control/acrobot-swingup_sparse-v0", render_mode="human")
Run the environment:
observation, info = env.reset(seed=42)
for _ in range(1000):
action = env.action_space.sample() # this is where you would insert your policy
observation, reward, terminated, truncated, info = env.step(action)
if terminated or truncated:
observation, info = env.reset()
env.close()
To get a list of all available dm_control
environments (85 total):
from gymnasium.envs.registration import registry
DM_CONTROL_ENV_IDS = [
env_id
for env_id in registry
if env_id.startswith("dm_control") and env_id != "dm_control/compatibility-env-v0"
]
print(DM_CONTROL_ENV_IDS)
Class Description¶
- class shimmy.dm_control_compatibility.DmControlCompatibilityV0(env: composer.Environment | control.Environment | dm_env.Environment, render_mode: str | None = None, render_kwargs: dict[str, Any] | None = None)[source]¶
This compatibility wrapper converts a dm-control environment into a gymnasium environment.
Dm-control is DeepMind’s software stack for physics-based simulation and Reinforcement Learning environments, using MuJoCo physics.
Dm-control actually has two Environments classes, dm_control.composer.Environment and dm_control.rl.control.Environment that while both inherit from dm_env.Environment, they differ in implementation.
For environment in dm_control.suite are dm-control.rl.control.Environment while dm-control locomotion and manipulation environments use dm-control.composer.Environment.
This wrapper supports both Environment class through determining the base environment type.
Note
dm-control uses np.random.RandomState, a legacy random number generator while gymnasium uses np.random.Generator, therefore the return type of np_random is different from expected.
Initialises the environment with a render mode along with render information.
Note: this wrapper supports multi-camera rendering via the render_mode argument (render_mode = “multi_camera”)
For more information on DM Control rendering, see https://github.com/deepmind/dm_control/blob/main/dm_control/mujoco/engine.py#L178
- Parameters:
env (Optional[composer.Environment | control.Environment | dm_env.Environment]) – DM Control env to wrap
render_mode (Optional[str]) – rendering mode (options: “human”, “rgb_array”, “depth_array”, “multi_camera”)
render_kwargs (Optional[dict[str, Any]]) – Additional keyword arguments for rendering. For the width, height and camera id use “width”, “height” and “camera_id” respectively. See the dm_control implementation for the list of possible kwargs, https://github.com/deepmind/dm_control/blob/330c91f41a21eacadcf8316f0a071327e3f5c017/dm_control/mujoco/engine.py#L178 Note: kwargs are not used for human rendering, which uses simpler Gymnasium MuJoCo rendering.
- metadata: dict[str, Any] = {'render_fps': 10, 'render_modes': ['human', 'rgb_array', 'depth_array', 'multi_camera']}¶
- property dt¶
Returns the environment control timestep which is equivalent to the number of actions per second.
- reset(*, seed: int | None = None, options: dict[str, Any] | None = None) tuple[ObsType, dict[str, Any]] [source]¶
Resets the dm-control environment.
- step(action: ndarray) tuple[ObsType, float, bool, bool, dict[str, Any]] [source]¶
Steps through the dm-control environment.
- property np_random: RandomState¶
This should be np.random.Generator but dm-control uses np.random.RandomState.